Data Mesh vs Data Lake: How They Impact Cyber Security
- Company Contributor
It’s the age of self-service business intelligence (BI), and nearly every enterprise considers itself a data-first organization. Unfortunately, many don’t treat their data architecture with a high level of democratization and scalability.
So what’s the right way to manage your growing volumes of enterprise data and still provide the data quality, governance, and consistency required for analytics at scale?
Do you centralize your data in a data lake or opt for a distributed data mesh architecture?
And how can you ensure cybersecurity compliance while still maintaining efficiency?
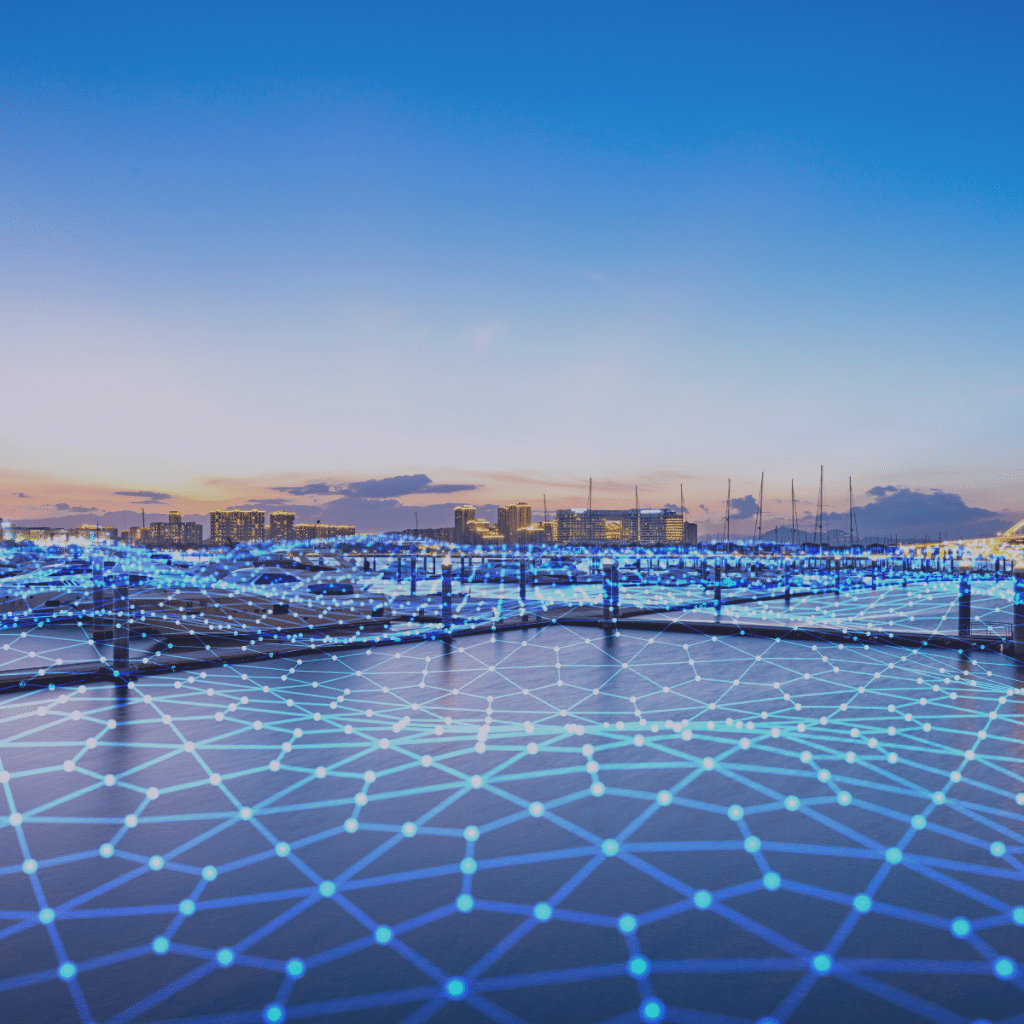
The Data Management Solutions Organizations are Taking
Most organizations want to analyze data efficiently without transforming or moving it through complicated extract, transform, and load (ETL) processes.
Many organizations face a shortage of skilled data science, data engineering, and analyst talent. IT teams often rely on existing infrastructure, spending a lot of their time modeling and transforming data so that users can analyze and use it.
However, this approach is problematic because it’s impossible to interrogate data severally without going back to the engineering or centralized data science team to transform the data again. It makes the entire data management approach unsustainable across multiple business domains.
As a result, many IT and data leaders are trying to upskill employees to become citizen data scientists. In the process, they have launched self-service business intelligence (BI) and data literacy initiatives to help enterprise users use analytics to drive smarter decisions.
However, success relies on the premise that businesses must empower their employees and users to analyze and query data where it lives.
Which approach is better between centralizing data in a data lake and distributing it across various data meshes? Let’s first look at the two systems and their pros and cons before determining which enterprise data management strategy best suits your team.
What is a Data Lake?
A data lake is a storage repository organizations use to centralize, organize, and protect unstructured, structured, and semi-structured data from multiple sources.
Data lakes use a schema-on-read approach, meaning they structure data at query-time based on users’ needs. In comparison, data warehouses follow a schema-on-write approach and structure data as it enters the warehouse.
Data lake storage solutions are increasingly popular mainly because of cloud object storage. However, it’s essential to note that they do not include analytic features.
Instead, organizations and third-party service providers often combine data lakes with other cloud-based services and use downstream software tools for indexing, querying, transformation, analytics, and cybersecurity.
A data lake architecture is characterized by a self-service data analytics engine sitting atop a cloud-based data repository to deliver key features to help organizations realize their data’s value and utility.
The approach can activate low-cost public cloud object storage, such as Google Cloud or Amazon S3, enabling teams to ingest, analyze, and index data without first moving it into separate ETL pipelines for analysis.
Pros of a Data Lake
Below are the benefits of a data lake:
- Data democratization: Data lakes make data available to the entire organization instead of the top leadership alone. It reduces bureaucracy and allows staff to make informed decisions quickly at their level.
- Simplicity: Data lakes ingest various types of data, eliminating the need for data modeling at storage. Instead, organizations can filter and model data when the need arises.
- Scalability: This allows for relatively inexpensive scalability than traditional data warehouses.
- Flexibility: A data lake allows organizations to become schema-free or define multiple schemas, which is excellent for analytics.
- Versatility: Data lakes can store multi-structured data, including logs, multimedia, XML, binary, chat, social data, sensor data, or people data.
- Simple Data Platform: Centralizes data storage on a single platform.
Cons of a Data Lake
Below are the benefits of a data lake:
- Complex on-premises deployment: Deploying an on-premises data lake is a significantly complex process, and it’s easier to deploy them in the cloud.
- Learning curve: Data lakes have a long learning curve with new tools and services. It could require training, outsourcing, or recruiting team members with rare data skills.
- Migration: Transitioning to and from a data lake is challenging and requires careful planning and execution to manage your data sets.
- Increased security risks: Centralized data systems have increased security risks and access control problems. Additionally, poor oversight could lead to unauthorized data access.
What is a Data Mesh?
Data mesh refers to an architectural design that tackles the challenges associated with distributed and decentralized data. So instead of a centralized data lake, data meshes allow the access of multiple disparate data sources through an abstraction layer.
Under a data mesh approach, organizations federate data ownership to business domains that assume responsibility for security and governance – instead of a single IT team tasked with managing an entire data lake.
The individual data product owners work collaboratively and oversee their specific data to drive governance consistency.
Essentially, it’s data governance decentralization in tandem with data decentralization while still enabling centralized guardrails.
A data mesh architecture connects many data sources into a coherent infrastructure, making data accessible to those with authorized access. Ideally, a data mesh approach allows users to interrogate data numerous times without moving and transforming it again.
Pros of a Data Mesh
Data mesh architecture is a paradigm shift from traditional data platform management. It moves from centralized, unified data platforms and their shortcomings towards decentralized, independent, and efficient domain teams.
Some of its benefits include:
- Domain teams have independence over technology and prioritization that fits their needs
- It views data-as-a-product and supports data interoperability, reducing IT backlog and enabling business teams to independently operate while focusing on data products relevant to their specific needs.
- Data Mesh overcomes data residency concerns under regulations such as GDPR because it acts as a connectivity layer. It means that an American company can access data residing in the European Union because data mesh enables direct access and querying without physically moving data into the US.
- A data mesh avoids high bandwidth data transfers that significantly increase the cost of cloud service platforms.
- Data mesh improves business domain agility and scalability.
- It leads to faster data delivery by using a self-service approach to making data accessible to authorized consumers and hiding the underlying complexities.
Cons of a Data Mesh
Despite having numerous benefits for organizations, data mesh has a few challenges.
These include:
- Data mesh architecture faces complexities due to managing multiple data products across various autonomous domains.
- Multi-domain data duplication and redundancy occur when data from one domain gets repurposed to serve another domain’s enterprise needs, potentially impacting resource utilization and increasing data management costs.
- Challenges stemming from jurisdictional data governance and quality assurance – for example, domains may have different governance and quality requirements that an organization must consider when sharing data products and pipelines.
- Domain experts may lack the skills of using specific domain programming languages the architecture uses.
- Many data mesh programs are not API compatible, making it difficult for some enterprises to complete their required tasks.
- Organizations must define an enterprise-wide data model to consolidate various data products, making them available to authorized users in a central location.
Data Mesh vs Data Lake: Which One is Right for Your Organization?
So which should you pick in the data mesh vs data lake decision process?
Data lakes are great for organizations looking for a centralized system for all their data needs. However, their poor scalability and agility are huge setbacks.
On the other hand, data mesh architecture gives users more control, but since data has various uses, a centralized system makes sense for efficiently complementing data transformations.
One could say that data lakes suit smaller organizations, but giant corporations require a data mesh system to speed up their data processes through autonomy and flexibility. It saves teams a lot of time, resulting in a competitive edge.
Ultimately, it is futile to compare these two distinctive data architectures because they are conceptually different. Data lakes are centralized data storage repositories that store, organize and protect data, while data mesh architectures are principles for decentralized data management.
The primary objective for both is offering organizations faster analytical insights and increasing the business value of analytics. Organizations distribute and query data mesh systems from domain-owned data storage, including data lakes. Therefore, data lakes and meshes can coexist and complement each other.
Whatever approach an organization takes must include data security by design. It must ensure data encryption (at rest and in motion) and masking to meet data privacy regulations.
Data security by design ensures organizations achieve data security so that cybersecurity does not become an afterthought but part of the software development process.
The Bottom Line
So is the question of data mesh vs data lake important? Not really, because data lakes and meshes can coexist since they complement each other.
Many organizations double down on a data lake approach with cloud infrastructure while others store data across multiple locations – on-premises and cloud-based. A data mesh architecture can also work if one of those endpoints is a cloud data lake.
The desired end state for organizations is having a unified platform for analytics. Additionally, users want ready access to data and the ability to analyze it where it resides without using complex data modeling and engineering.
As a result, organizations are trying new approaches to democratize data access by leveraging their cloud storage and computing assets.
Explore More Resources
- Article, News
- Blog
- Blog
- Blog
Your Trusted Source for Cyber Education
Sign up for ThriveDX's quarterly newsletter to receive information on the latest cybersecurity trends, expert takes, security news, and free resources.